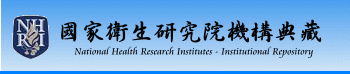 |
English
|
正體中文
|
简体中文
|
Items with full text/Total items : 12145/12927 (94%)
Visitors : 904722
Online Users : 535
|
|
|
Loading...
|
Please use this identifier to cite or link to this item:
http://ir.nhri.org.tw/handle/3990099045/14321
|
Title: | Discrimination of methicillin-resistant staphylococcus aureus by MALDI-TOF mass spectrometry with machine learning techniques in patients with staphylococcus aureus bacteremia |
Authors: | Kong, PH;Chiang, CH;Lin, TC;Kuo, SC;Li, CF;Hsiung, CA;Shiue, YL;Chiou, HY;Wu, LC;Tsou, HH |
Contributors: | Institute of Population Health Sciences;National Institute of Infectious Diseases and Vaccinology |
Abstract: | Early administration of proper antibiotics is considered to improve the clinical outcomes of Staphylococcus aureus bacteremia (SAB), but routine clinical antimicrobial susceptibility testing takes an additional 24 h after species identification. Recent studies elucidated matrix-assisted laser desorption/ionization time-of-flight mass spectra to discriminate methicillin-resistant strains (MRSA) or even incorporated with machine learning (ML) techniques. However, no universally applicable mass peaks were revealed, which means that the discrimination model might need to be established or calibrated by local strains' data. Here, a clinically feasible workflow was provided. We collected mass spectra from SAB patients over an 8-month duration and preprocessed by binning with reference peaks. Machine learning models were trained and tested by samples independently of the first six months and the following two months, respectively. The ML models were optimized by genetic algorithm (GA). The accuracy, sensitivity, specificity, and AUC of the independent testing of the best model, i.e., SVM, under the optimal parameters were 87%, 75%, 95%, and 87%, respectively. In summary, almost all resistant results were truly resistant, implying that physicians might escalate antibiotics for MRSA 24 h earlier. This report presents an attainable method for clinical laboratories to build an MRSA model and boost the performance using their local data. |
Date: | 2022-05-16 |
Relation: | Pathogens. 2022 May 16;11(5):Article number 586. |
Link to: | http://dx.doi.org/10.3390/pathogens11050586 |
JIF/Ranking 2023: | http://gateway.webofknowledge.com/gateway/Gateway.cgi?GWVersion=2&SrcAuth=NHRI&SrcApp=NHRI_IR&KeyISSN=2076-0817&DestApp=IC2JCR |
Cited Times(WOS): | https://www.webofscience.com/wos/woscc/full-record/WOS:000803182300001 |
Cited Times(Scopus): | https://www.scopus.com/inward/record.url?partnerID=HzOxMe3b&scp=85130682180 |
Appears in Collections: | [鄒小蕙] 期刊論文 [邱弘毅] 期刊論文 [熊昭] 期刊論文 [郭書辰] 期刊論文
|
Files in This Item:
File |
Description |
Size | Format | |
PUB35631107.pdf | | 2188Kb | Adobe PDF | 203 | View/Open |
|
All items in NHRI are protected by copyright, with all rights reserved.
|