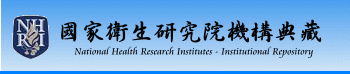 |
English
|
正體中文
|
简体中文
|
Items with full text/Total items : 12145/12927 (94%)
Visitors : 904033
Online Users : 798
|
|
|
Loading...
|
Please use this identifier to cite or link to this item:
http://ir.nhri.org.tw/handle/3990099045/15626
|
Title: | A machine learning-based ensemble model for estimating diurnal variations of nitrogen oxide concentrations in Taiwan |
Authors: | Asri, AK;Lee, HY;Chen, YL;Wong, PY;Hsu, CY;Chen, PC;Lung, SC;Chen, YC;Wu, CD |
Contributors: | National Institute of Environmental Health Sciences |
Abstract: | Air pollution is inextricable from human activity patterns. This is especially true for nitrogen oxide (NO(x)), a pollutant that exists naturally and also as a result of anthropogenic factors. Assessing exposure by considering diurnal variation is a challenge that has not been widely studied. Incorporating 27 years of data, we attempted to estimate diurnal variations in NO(x) across Taiwan. We developed a machine learning-based ensemble model that integrated hybrid kriging-LUR, machine-learning, and an ensemble learning approach. Hybrid kriging-LUR was performed to select the most influential predictors, and machine-learning algorithms were applied to improve model performance. The three best machine-learning algorithms were suited and reassessed to develop ensemble learning that was designed to improve model performance. Our ensemble model resulted in estimates of daytime, nighttime, and daily NO(x) with high explanatory powers (Adj-R(2)) of 0.93, 0.98, and 0.94, respectively. These explanatory powers increased from the initial model that used only hybrid kriging-LUR. Additionally, the results depicted the temporal variation of NO(x), with concentrations higher during the daytime than the nighttime. Regarding spatial variation, the highest NO(x) concentrations were identified in northern and western Taiwan. Model evaluations confirmed the reliability of the models. This study could serve as a reference for regional planning supporting emission control for environmental and human health. |
Date: | 2024-03-15 |
Relation: | Science of the Total Environment. 2024 Mar 15;916:Article number 170209. |
Link to: | http://dx.doi.org/10.1016/j.scitotenv.2024.170209 |
JIF/Ranking 2023: | http://gateway.webofknowledge.com/gateway/Gateway.cgi?GWVersion=2&SrcAuth=NHRI&SrcApp=NHRI_IR&KeyISSN=0048-9697&DestApp=IC2JCR |
Cited Times(WOS): | https://www.webofscience.com/wos/woscc/full-record/WOS:001176422200001 |
Cited Times(Scopus): | https://www.scopus.com/inward/record.url?partnerID=HzOxMe3b&scp=85183450392 |
Appears in Collections: | [陳裕政] 期刊論文 [陳保中] 期刊論文
|
Files in This Item:
File |
Description |
Size | Format | |
PUB38278267.pdf | | 11149Kb | Adobe PDF | 116 | View/Open |
|
All items in NHRI are protected by copyright, with all rights reserved.
|